Explainable AI: Why business leaders should care
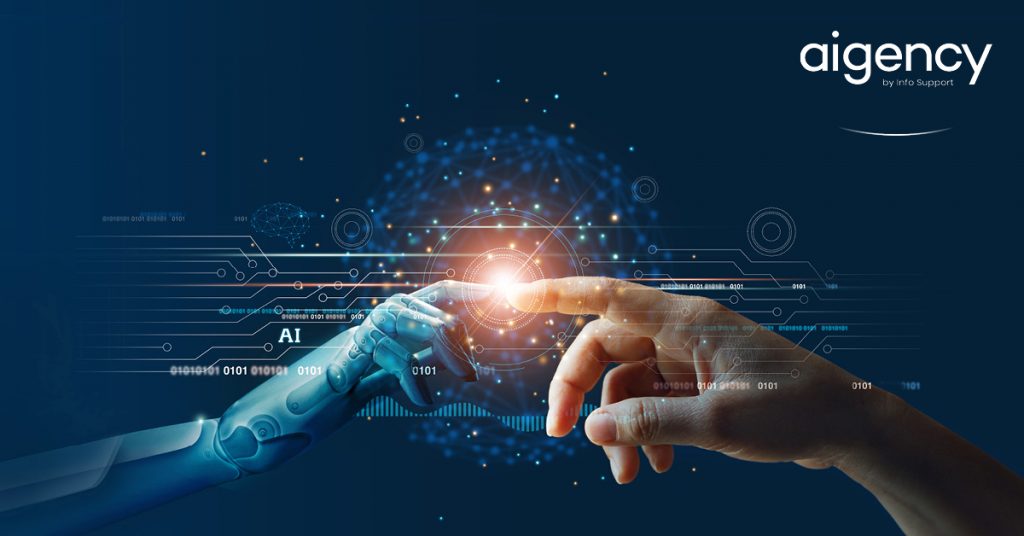
Imagine sitting across from your doctor as she delivers unsettling news. She informs you that you have a high risk of dying within the next two weeks. Naturally, your immediate response might be to seek solace in a nearby pub. However, instead of drowning your sorrows, consider how to lower this risk.
This scenario is based on a machine learning model developed for thrombosis patients. The model predicts the likelihood of severe bleeding within two weeks, considering various factors such as medication and medical history. As we can all agree, knowing the accuracy of this prediction is not enough. What truly matters is understanding how we can act upon this information to reduce the risk of fatality.
Enter the concept of explainability.
Explanations are essential for both life-or-death situations and business applications. Whether we’re dealing with predicting customer churn or identifying equipment failures, understanding the underlying factors behind the predictions is crucial. And that is why business leaders should care about Explainable AI.
Explainable AI, or XAI, refers to the ability of AI systems to provide understandable explanations for their decision-making processes. Unlike traditional “black-box” models that operate as obscure entities, Explainable AI empowers leaders with the tools to comprehend, interpret, and validate the decisions made by AI systems. This transparency not only fosters trust but also enables organizations to meet regulatory requirements, mitigate risks, and identify and rectify biases or flaws in AI algorithms.
Explainable AI empowers leaders with the tools to comprehend, interpret, and validate the decisions made by AI systems.
The benefits of embracing Explainable AI go beyond mere transparency. Business leaders who prioritize explainability gain a better understanding of potential biases that can unintentionally influence decision-making processes. By comprehending the factors that drive AI predictions and recommendations, leaders can ensure that ethical considerations are woven into the fabric of their AI systems. This, in turn, helps organizations avoid reputational damage and legal consequences stemming from biased or discriminatory outcomes.
Now, let’s delve into the concept of transparency by exploring the distinction between “black-box” and “glass-box” or “white-box” models.
Black, glass and white-box models.
“Black-box” models, as the name suggests, conceal their inner workings from users. They provide predictions without revealing the underlying reasoning behind them. In contrast, “glass-box” or “white-box” models offer varying levels of transparency and interpretability. A white-box model, also known as a transparent or interpretable model, provides a clear understanding of its internal workings. It presents explicit rules or equations that govern its predictions, allowing users to easily trace and comprehend the decision-making process. Linear regression and decision trees are examples of white-box models that prioritize interpretability, enabling users to validate and explain the model’s outputs.
Glass-box models, on the other hand, strike a balance between interpretability and performance. They offer some visibility into their decision-making process, providing intermediate explanations or high-level insights. While not as easily interpretable as white-box models, glass-box models, such as certain types of neural networks, can still offer feature importance rankings or highlight relevant patterns in the data. These models cater to scenarios where a higher degree of accuracy is required while still providing some level of explanation.
Both white-box and glass-box models contribute to the goals of Explainable AI by offering varying degrees of transparency and interpretability. The choice between the two depends on the specific requirements of the problem at hand, the need for interpretability, and the desired trade-off between model complexity and performance.
Pitfalls and a clever horse.
When we explore the potential pitfalls of machine learning models it becomes even more clear why understanding their reasoning is crucial.
Machine learning models excel at identifying patterns in data. However, we must ensure that they learn the correct patterns. Otherwise, they may provide accurate predictions for the wrong reasons. This phenomenon is not new; it dates back to the late 1800s and early 1900s.
During that time, there was a horse named Clever Hans who astounded people with his ability to perform intellectual tasks such as arithmetic. Crowds would gather to witness the horse tapping his hoof to indicate the correct answer to mathematical questions posed by his owner.
However, the truth behind Clever Hans’s intelligence was not what it seemed. It was later discovered that the horse was not actually performing complex calculations or understanding language. Clever Hans was clever indeed but in a different way. He was responding to involuntary cues in the body language of his owner, who was unaware that he was providing subtle signals to guide the horse’s responses.
Clever Hans was clever indeed but in a different way.
This fascinating anecdote serves as a reminder of the risks inherent in machine learning models. These models can learn something and provide accurate predictions, but their reasoning may be based on incorrect or unintended cues.
Let me provide you with a couple of real-life examples.
One famous case involved a machine learning model that classified images of sheep based on the presence of a green background. While the model achieved high accuracy during training, its performance in real-life scenarios was abysmal. The reason? The model learned to associate sheep with the color green rather than the actual features of sheep. Such reliance on misleading cues can lead to errornuous outcomes when deployed in the real world.
In another instance of more importance, a machine learning model was developed to detect lung cancer in X-ray images. The model’s accuracy during training was impressive, yet its performance in practice was highly unreliable. Upon investigation, it was discovered that the model had learned to interpret the scribbles made by radiologists on the X-ray images, rather than focusing on the actual signs of lung cancer. As you can imagine, this led to not only erroneous but also dangerous predictions.
These examples underscore the importance of knowing how machine learning models reason and make predictions. It is not enough to rely solely on accuracy metrics. We must strive to understand the underlying factors and logic that drive the model’s decisions.
The disagreement problem.
Even an additional challenge arises with glass-box models—the issue of the disagreement problem.
Glass-box models utilize a surrogate model to gain insight into the reasoning of a black-box model. This surrogate model attempts to understand the decision-making process by comparing differences between inputs and outputs. However, because it is an approximation of the black-box model, there is always a risk of disagreement between the two.
Also, different methods for training surrogate models can yield varying explanations and reasoning for the black-box model. This disagreement poses a challenge to reliably understanding the inner workings of the black-box model despite attempts to create a glass-box representation.
So, how can we address these challenges and make machine learning models more interpretable and transparent? The answer lies in interpretable machine learning models.
Interpretable machine learning models.
Interpretable machine learning models, the white-box models, are characterized by their inherent transparency and human readability. These models, such as decision trees, linear regression, or rule-based models, produce outputs that users can easily understand. They provide explicit rules, equations, or feature importance rankings that explain how they arrive at their predictions.
The transparency of interpretable models allows users to trace the decision path, identify influential factors, and validate the reliability of the outputs. By instilling trust and understanding, these models facilitate collaboration between humans and machines. They also aid in regulatory compliance by providing transparent and interpretable insights that can be audited and understood by external entities.
Fortunately, there is a growing availability of interpretable algorithms that can be employed in various applications. Many of these algorithms, such as the Explainable Boosting Machine and ProtoPNet, are relatively unknown and underutilized by data science teams.
There is a growing availability of interpretable algorithms that can be employed in various applications.
Allow me to provide you with an example of an interpretable computer vision model called ProtoPNet. This deep learning model for computer vision offers interpretable explanations directly from the model itself. It utilizes prototypes of trained images, which can be compared to the classified image in a “this looks like that” manner. ProtoPNet highlights similar features, such as beaks, feathers, colors, and structures, and allows users to compare them with the corresponding features in the classified image. By making these visual comparisons, it becomes easy to understand why the model predicted a certain class for the image. ProtoPNet demonstrates that interpretability in computer vision models can be achieved without necessarily relying on glass-box or black-box approaches.
White-box over black-box models.
I firmly believe it’s essential that business leaders must prioritize interpretable machine learning models and consider them as preferred alternatives over white-box and black-box models.
First and foremost, interpretable models enhance trust and transparency in AI-driven decision-making processes. By understanding how the model arrives at its predictions, business leaders can have greater confidence in the reliability and fairness of the outcomes. This is crucial for building trust among stakeholders and ensuring ethical considerations are upheld in decision-making.
Secondly, interpretable models offer more precise insights into the factors driving predictions. The explicit rules, equations, or feature importance rankings provided by interpretable models enable leaders to identify the key variables influencing outcomes. This empowers them to make more informed business decisions and take appropriate actions based on these insights.
Moreover, interpretable models facilitate regulatory compliance. They provide explanations that can be audited and understood by external entities, helping organizations meet legal requirements that demand transparency and accountability in decision-making. By embracing interpretable models, businesses can mitigate potential legal risks and ensure regulatory compliance.
Interpretable models foster collaboration between business leaders and data scientists.
Furthermore, interpretable models foster collaboration between business leaders and data scientists. These models’ clear and understandable nature allows leaders to actively participate in discussions about model development, ask relevant questions, and contribute their domain expertise. This collaboration leads to improved model performance and more effective decision-making overall.
Lastly, interpretable models help mitigate potential biases and discrimination. By providing visibility into the decision-making process, leaders can identify and rectify biases or unfairness in the models. This promotes fairness and inclusivity within organizations, avoiding reputational damage and legal consequences arising from biased or discriminatory outcomes.
Business leaders should prioritize interpretable machine learning models because they enhance trust, support informed decision-making, facilitate regulatory compliance, foster collaboration, and mitigate bias. By embracing interpretability, leaders can effectively leverage AI technologies while ensuring transparency, fairness, and ethical considerations are at the forefront of their business operations.
Learn more.
If you’d like to learn more about how we, as Aigency, can help you open up your black-box models and extract real value from interpretable machine-learning models, I invite you to contact us at aigency.com and discuss how we can help you. Together, we can harness the power of Explainable AI and drive meaningful and responsible innovation in your organization.
Author
By Joop Snijder, CTO Aigency
With over a decade of experience in AI, he has developed a passion for explainable and interpretable AI, helping businesses harness the power of this cutting-edge technology to drive innovation and growth.